MLOps : A new Boom in the AI world
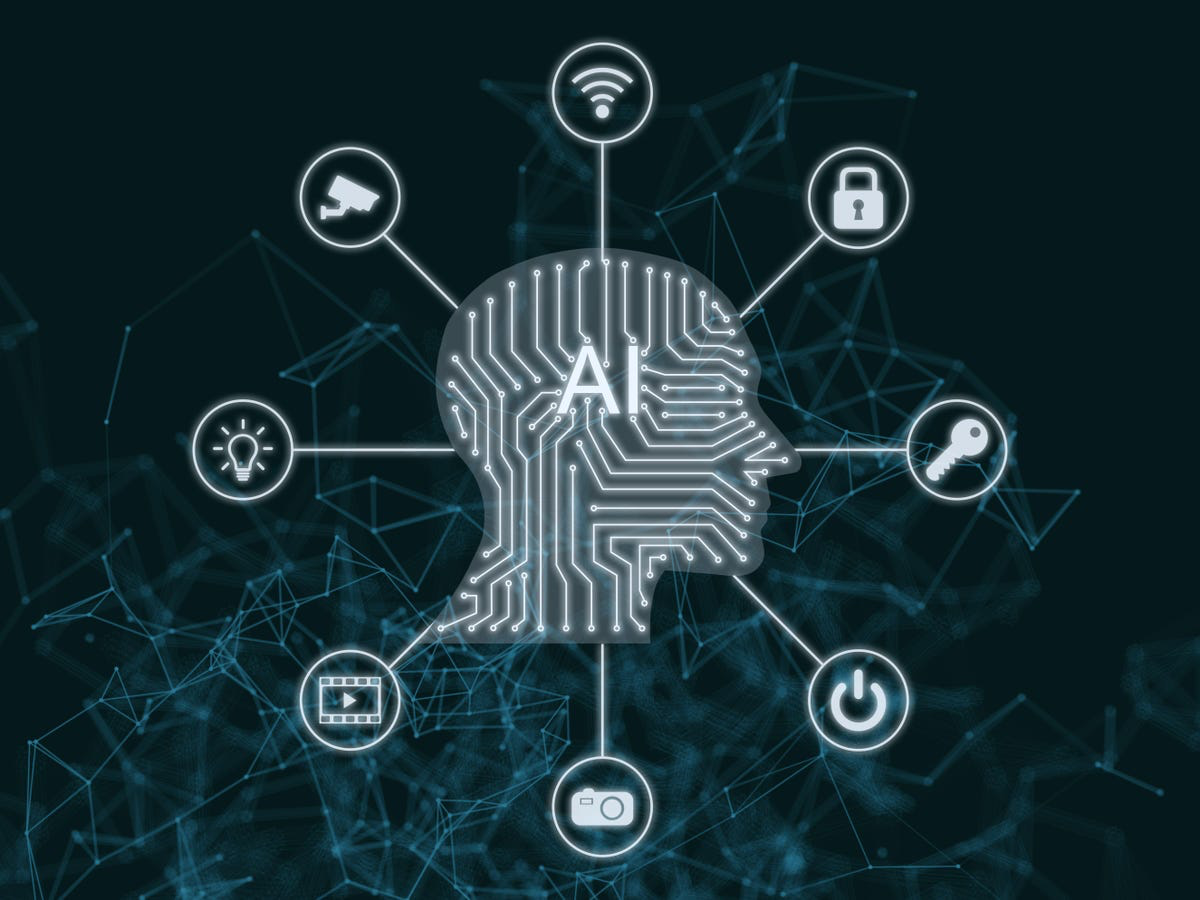
Machine Learning Operations (MLOps) is a vital technology for scaling out machine learning since it is a missing piece in the business AI puzzle. This is the key driver behind MLOps, a novel concept in the domain of AI (Artificial Intelligence).
There is too much disagreement about the definition, as different people have different opinions.
MLOps is an expression for Machine Learning Operations/ Machine Learning Engineering for Production, a core function of Machine Learning engineering that focuses on simplifying the process of delivering machine learning models to production, in addition to maintaining and monitoring them. However, in layman's terms, it is the notion of taking a model and generating a product and service out of it, while all of the challenges that arise when we attempt to do so are the job of MLOps.It provides a collaborative platform that allows data scientists and systems engineers to work together to design, implement, and monitor models successfully.
An MLOps automates the operational and synchronization aspects of the machine learning lifecycle.
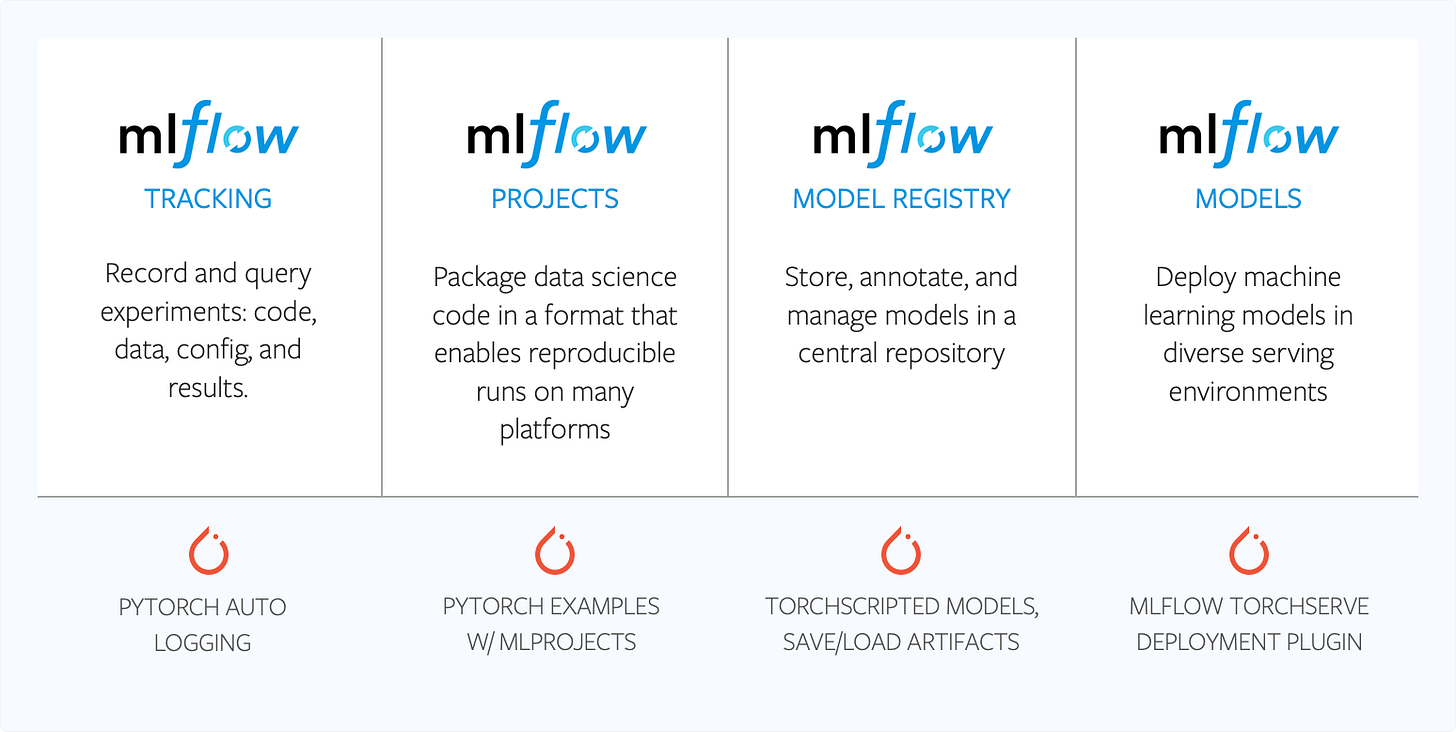
So all the above we talked about MLOps, But we find it is somewhere related to DevOps.Wondering How”
MLOps (the data-driven brother of DevOps).
"MLOps is the natural progression of DevOps in the context of AI,” said Samir Tout, a Professor of Cybersecurity at the Eastern Michigan University's School of Information Security & Applied Computing (SISAC).
Then what's the difference? DevOps brings a rapid, continuously iterative approach to shipping applications; MLOps is a set of engineering practices specific to machine learning projects that borrows the same principles to take machine learning models to production. From both, the outcome is higher software quality and cu’tomer satisfaction.
While it leverages DevOps' focus on security, com’liance, and management of IT resources, MLOps’ real emphasis is on the consistent and smooth development of models and their scalability.
The origins”of MLOps start in 2015 from a paper entitled “Hidd”n Technical Debt in Machine Learning robust tthe growth has been particularly strong, just like from a fetus to a healthy child of AI.
It closes the gap between existing software delivery infrastructures, DevOps engineers, Machine Learning developers, training platforms, and data scientists, permitting machine learning models to be delivered faster.
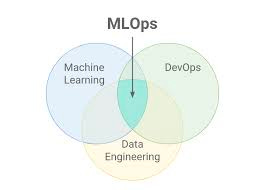
According to Gartner, MLOps is a subset of ModelOps. MLOps focuses on the operationalisation of ML models, while ModelOps covers all types of AI models.
Then what are the components of MLOps? And most importantly, how does MLOps work?
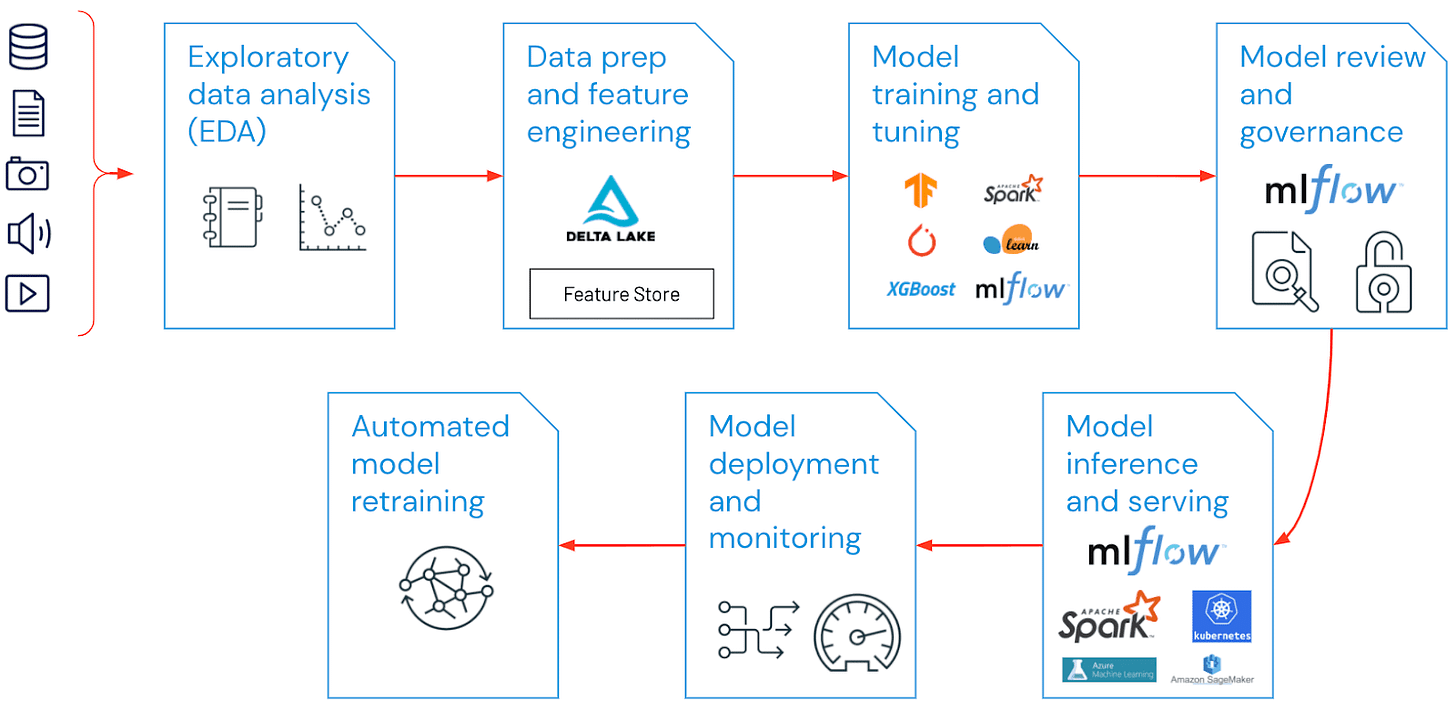
In machine learning projects, the range of MLOps can be as narrow or broad as the project requires. MLOps can sometimes include everything from the data pipeline to model production, while other projects might simply need to apply MLOps for the model distribution process.
The stagewhat'sich MLOps principles are being employed can be used to distinguish the best MLOps approaches.
So what’s the use of all this? Why are we taking this so seriously?
MLOps will assist in the creation and quality of machine learning and AI solutions. By establishing continuous integration and deployment methods with proper monitoring, validation, and governance of Machine learning, scientists and machine learning engineers can collaborate and accelerate model development and production by employing an MLOps strategy.
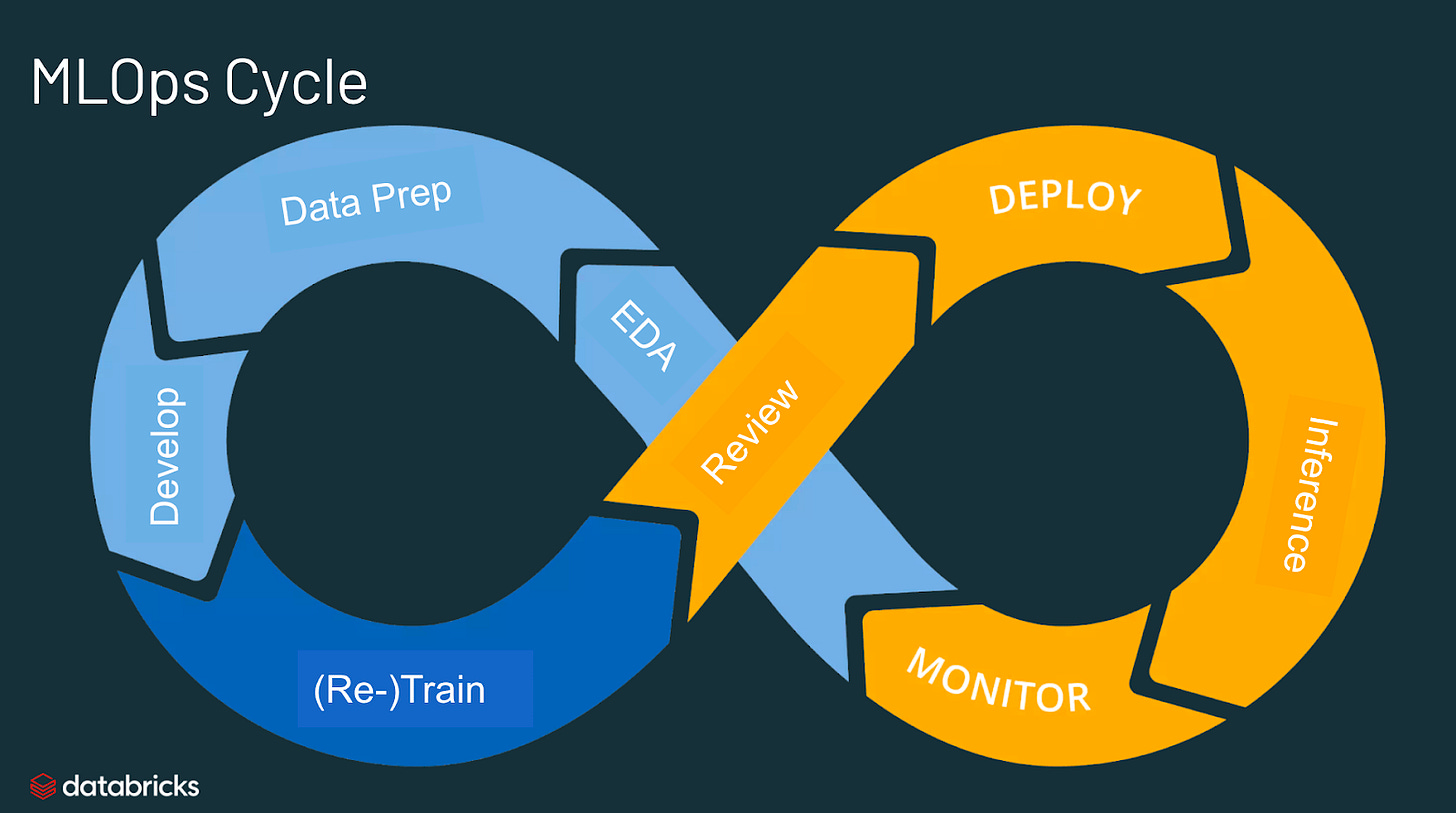
Many complicated components comprise the machine learning pipeline, including data import, data preparation, model training, model tuning, model deployment, model monitoring, explainability, and many more. It also necessitates cross-team communication and hand-offs, from Data Engineering to Data Science to Machine learning Engineering.
To maintain all of these processes synchronized and running in unison, high operational rigor is required which can be easily done by the help of MLOps.
MLOps brings together experts from many fields and ideas to address a shared challenge.
Are there any benefits?
The key advantages of MLOps in building Machine Learning Models are efficiency, scalability, and risk reduction.
According to McKinsey, by 2030, Machine Learning could add up to 13 trillion dollars back into the global economy by enabling workers in all sectors to improve their output.
According to IBM, by 2023, 70% of AI workloads will be deployed using application containers or a serverless programming approach, necessitating a DevOps culture. Furthermore, Algorithmia estimates that 85% of machine learning models are never delivered. Firms have challenges in developing machine learning applications, managing such models, and putting all into action.
To solve these challenges, other companies, such as DataRobot, have emerged as the sector's top machine learning operations tool enablers.
Let’s come to the main topic, Does MLOps drive business growth?
It is extremely difficult to operationalize and scale machine learning to produce commercial value.
Indeed, it is now rather simple to understand enough about machine learning and AI to be hazardous. It is, nevertheless, all too simple to be harmful. This is because, although building models has become simpler, converting them from scientific projects into dependable, scalable software that adds value to businesses remains difficult.
“Some key best practices are having a reproducible pipeline for data preparation and training, having a centralized experiment tracking system with well-defined metrics, and implementing a model management solution that makes it easy to compare alternative models across various metrics and roll back to an old model if there is a problem in production,” said Matei Zaharia, who is the chief technologist at Databricks.
These tools make it easy for Machine Learning teams to understand the performance of new models and catch and repair errors in production.
"Monitoring/testing of models requires a clear understanding of the data biases," said Michael Berthold, who is the CEO and co-founder of KNIME.
Majority of the solutions can be found in scientific studies on the event, model change, and drift detection, but they are typically overlooked in practice. You must test on independent data, use competitor models, and recalibrate frequently. Most data science toolboxes today completely disregard this component and have a very restricted perspective on 'end-to-end' data science.
We now demand the software on which we base our businesses to be scalable, dependable, and efficient. And, to gain the benefits of AI, the models that increasingly drive business choices will need to be the same. DevOps has optimized the way we develop, run, and maintain software for years, and it's now time to do the same for machine learning. This is unfamiliar ground for many, but experts have learnt how to make AI function at scale via years of trial and error—with MLOps—and it will ensure the company gets the most out of its machine learning investments.
According to Forrester, over 50% of enterprise technology decision-makers have already implemented or are in the process of implementing Machine Learning and AI.
The Next Frontier Of Scaling AI In The Enterprise Is Leading MLOps Tools
It's past time for businesses to pay attention to MLOps and use it as a foundation for conducting machine learning initiatives.
MLOps combines the advantages of iterative development in machine learning model training with scalable and manageable model deployment. It is now the missing jigsaw component in corporate AI strategy.
The MLOps industry is still in its early stages, with technological solutions for efficient model management only appearing in the last year or two. However, the MLOps market is likely to exceed $4 billion in a few years, and as such, it promises to be a prime component of the AI solution landscape in the near future. Indeed, it has been predicted to be a prominent trend as early as 2020.
No doubt, MLOps is a long racehorse.
This article was originally published on the company blog.
Intellicy is a consultancy firm specialising in artificial intelligence solutions for organisations seeking to unlock the full potential of their data. They provide a full suite of services, from data engineering and AI consulting to comment moderation and sentiment analysis. Intellicy's team of experts work closely with clients to identify and measure key performance indicators (KPIs) that matter most to their business, ensuring that their solutions generate tangible results. They offer cross-industry expertise and an agile delivery framework that enables them to deliver results quickly and efficiently, often in weeks rather than months. Ultimately, Intellicy helps large enterprises transform their data operations and drive business growth through artificial intelligence and machine learning.